Bursting the p-value bubble
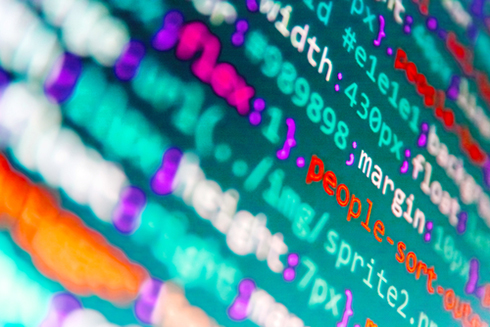
A vast amount of published bioscience research is based on flawed data analysis, and only a complete shift in priorities can reverse this dangerous situation
The Biologist Vol 64 (1) p7
Data analysis is an essential component of scientific work. However, it can be misapplied, misused and misinterpreted.
Most investigators in the biological sciences receive limited instruction on formal study design and data analysis, and those who are trained in it do not always keep track of new methodological improvements. Novel tools and platforms emerge quickly and they often have their own methodological peculiarities in order to be optimal, or even appropriate.
Well-trained biostatisticians are still a rare species and are involved as co-investigators and co-authors in only a modest segment of biological investigations. This may be even worse in biological research that does not involve human subjects.
Journals also lack the expertise required to understand and properly review statistics. Until a couple of years ago, even Nature and Science did not have trained statisticians routinely reviewing papers, which continues to be true of most biomedical and biology journals.
It is probably not surprising, then, that some spurious data analysis practices become copied and widely used across papers. Convenience, lack of expertise, lack of proper review oversight and inertia create large segments of literature that use these suboptimal or wrong methods.
In its extreme form, this phenomenon creates large bubbles containing huge numbers of papers affected by spurious methods. A 2016 paper showed that in fMRI neuroimaging studies, p-values of 0.05 obtained with common but inappropriate statistical methods[1] are actually often representing the equivalent of p-values of 0.9 or so. This means that thousands of papers may have claimed significant results while they were nowhere near any notion of significance.
There are many more examples across other disciplines, but perhaps the largest bubble is the suboptimal application and interpretation of p-values across biomedicine. Some 96% of papers that use p-values in biomedicine claim statistically significant results[2], an implausibly high rate of success. Moreover, in the few papers that do not report p<0.05, many find other analysis tools or manipulations to end up claiming significance of some sort anyway (a practice known as 'p-hacking').
In the majority of biomedical papers, the use of methods that lead to the computation of p-values and the rejection of hypotheses is a poor choice, and the interpretation of the results is even wrong. Other methods, such as Bayesian or false-discovery rate approaches, are often more appropriate because they address more directly the need to convey the strength of the evidence (how likely something is to be true or false). However, they are used less than 1% of the time in most fields.
Getting rid of the stereotypes and bubbles of poor data analysis is not an easy task. It requires a commitment from scientists, research institutions, journals, regulators and funders to enhance numeracy and analytical literacy in the scientific workforce.
Teams which publish scientific literature need a 'licence to analyse' and this licence should be kept active through continuing methodological education. This requires a shift in educational and research training priorities. Otherwise, we will continue to see many good ideas and interesting papers ruined by poor analysis. For biological research, and even more so for medical applications, this could eventually lead to deaths.
John Ioannidis is a professor of health research and co-director of the Meta-Research Innovation Center, Stanford University, California
References
1) Eklund, A. et al. Cluster failure: Why fMRI inferences for spatial extent have inflated false-positive rates. Proc. Natl. Acad. Sci. USA. 113(28), 7900–7905 (2016).
2) Chavalarias, D. et al. Evolution of reporting p-values in the biomedical literature, 1990–2015. JAMA 315(11), 1141–1148 (2016).